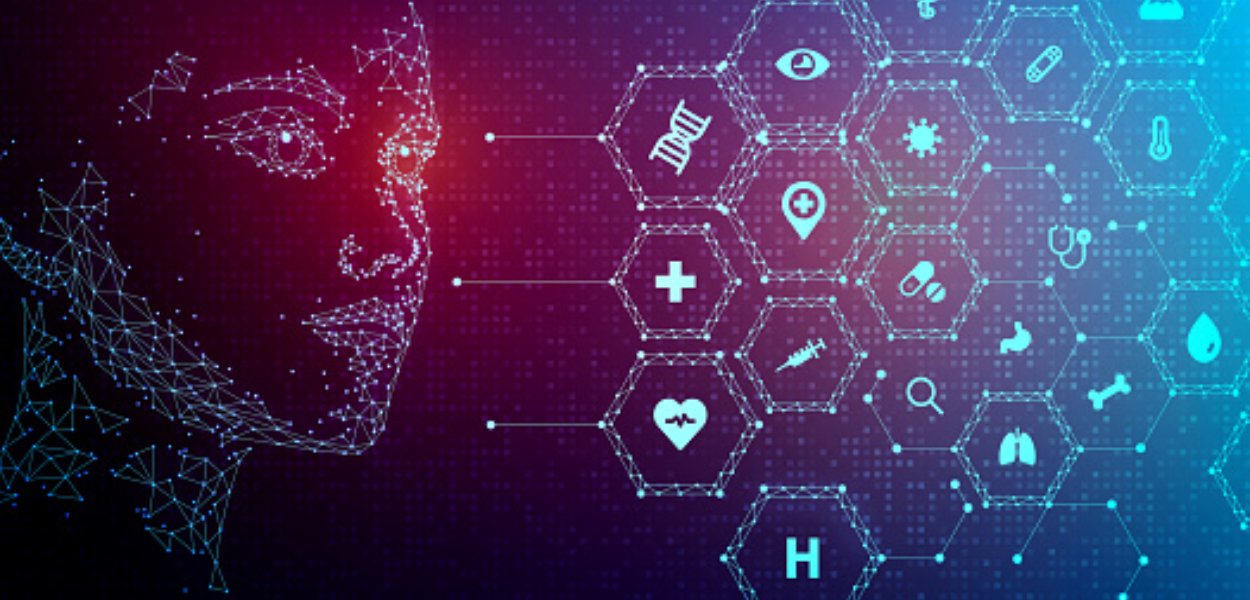
Blockchain’s Role in Enhancing AI Model Transparency and Trust
In the rapidly evolving landscape of artificial intelligence (AI), ensuring the transparency and trustworthiness of AI models has become paramount. The decisions and predictions made by AI systems affect various aspects of our lives, from finance to healthcare, and thus require a high level of scrutiny and accountability.
Blockchain technology, renowned for its transparency, security, and immutability, is emerging as a powerful tool to enhance the transparency and trust associated with AI models.
This intersection of blockchain and AI holds the potential to revolutionize how we build, deploy, and trust AI systems. This article delves into blockchain’s pivotal role in augmenting the transparency and trustworthiness of AI models, offering insights into the mechanisms and real-world applications that underscore this synergy.
Understanding AI Model Transparency and Trust
Transparency and trust are critical aspects of artificial intelligence (AI) models, ensuring their responsible and effective use in various domains. Here’s a closer look at what these terms mean in the context of AI:
Transparency
- Explainability: Transparency refers to understanding how an AI model arrives at its decisions or predictions. An AI system should provide clear and interpretable explanations for its outputs.
- Model Architecture: Transparency involves disclosing the architecture, algorithms, and data sources used in the AI model’s development. It should be clear how the model was trained and fine-tuned.
- Data Provenance: Transparent AI models maintain a record of the data used during training and testing, including its sources, quality, and potential biases.
- Algorithmic Transparency: It involves making the decision-making process of algorithms clear and comprehensible, especially in complex models like deep neural networks.
Trust
- Reliability: Trust in AI models hinges on their reliability and consistency. Users should have confidence that the model will produce accurate results under various conditions.
- Ethical Considerations: Trustworthy AI models adhere to ethical guidelines and principles, ensuring they do not discriminate, invade privacy, or produce biased outcomes.
- Security: Trust is linked to the protection of AI models. They should be protected against malicious attacks, data breaches, or adversarial manipulation.
- Accountability: Trustworthy AI models have mechanisms for accountability. Someone or something should be responsible for the model’s actions and a way to rectify errors or biases.
Achieving transparency and trust in AI models is vital not only for user acceptance but also for regulatory compliance and ethical considerations.
Emerging technologies like blockchain are being integrated into AI systems to enhance these aspects by providing secure, auditable, and transparent data management solutions. This combination can contribute significantly to AI’s responsible and trustworthy deployment in diverse applications.
Blockchain Technology Overview
Blockchain technology is a decentralized and distributed ledger system that has gained widespread recognition for its revolutionary capabilities. Here’s an overview of the key components and features of blockchain:
- Decentralization
- Distributed Ledger
- Immutability
- Cryptography
- Transparency
- Consensus Mechanisms
- Smart Contracts
Decentralization
- Blockchain operates on a network of computers (nodes) that are distributed across the globe. Unlike traditional centralized systems, there is no single controlling authority.
- Decentralization enhances security, eliminating single points of failure and reducing the risk of manipulation or fraud.
Distributed Ledger
- The blockchain ledger is a digital database that stores a record of transactions across multiple computers.
- Each block in the chain contains a set of transactions, and all blocks are linked together chronologically, forming a chain.
Immutability
- Once data is recorded on the blockchain, it becomes extremely difficult to alter or delete. This immutability is achieved through cryptographic hashing and consensus mechanisms.
- Immutability ensures data integrity, making blockchain suitable for applications where trust and security are paramount.
Cryptography
- Blockchain employs cryptographic techniques to secure data and transactions. Each block contains a cryptographic hash of the previous block, creating a secure link.
- Private and public keys are used to verify ownership and secure transactions.
Transparency
- All blockchain transactions are visible to participants in the network. This transparency fosters trust and accountability.
- While transactions are transparent, participants’ identities can be pseudonymous, depending on the blockchain’s design.
Consensus Mechanisms
- A consensus mechanism is used to validate and add new transactions to the blockchain. Common mechanisms include Proof of Work (PoW) and Proof of Stake (PoS).
- These mechanisms ensure that only legitimate transactions are added to the blockchain.
Smart Contracts
- Smart contracts are self-executing contracts with predefined rules and conditions. They automatically execute when conditions are met without the need for intermediaries.
- Ethereum, a popular blockchain platform, is known for supporting smart contracts.
Blockchain technology’s decentralized, transparent, and secure nature has the potential to transform industries by providing innovative solutions for data management, trust-building, and peer-to-peer transactions. Its applications continue to expand as developers and businesses explore its capabilities.
Blockchain’s Role in Enhancing AI Model Transparency
Blockchain can play a significant role in enhancing AI model transparency in several ways:
- Data Provenance and Traceability
- Model Training Verification
- Algorithmic Transparency
- Consensus Mechanisms for Model Evaluation
- Secure Data Sharing
- Proof of Work and Model Integrity
Data Provenance and Traceability
-
- Blockchain can securely record the entire data lifecycle, from collection to utilization. This provides an immutable and transparent audit trail of data sources and transformations.
- AI developers and users can trace back to the origin of data used for training AI models, ensuring data quality and highlighting potential biases or inaccuracies.
Model Training Verification
-
- By storing AI model training data and parameters on a blockchain, verifying that the model was trained on the intended data without tampering becomes possible.
- This ensures that AI models are not trained on unauthorized or compromised datasets, increasing trust in their results.
Algorithmic Transparency
-
- Blockchain can record the algorithms and parameters used in AI models. This transparency enables stakeholders to understand how decisions are made.
- Users can verify that AI models use ethical and compliant algorithms, reducing concerns about hidden biases.
Consensus Mechanisms for Model Evaluation
-
- Smart contracts on blockchain networks can be used to establish consensus mechanisms for evaluating AI models.
- These mechanisms can involve multiple parties, and decisions are recorded transparently on the blockchain, reducing the risk of biased evaluations.
Secure Data Sharing
-
- Blockchain facilitates secure and controlled data sharing among entities while maintaining data privacy and ownership.
- AI developers can access diverse datasets for model training, and data owners can ensure that their data is used transparently and ethically.
Proof of Work and Model Integrity
-
- In some cases, blockchain’s Proof of Work (PoW) or Proof of Stake (PoS) mechanisms can be used to validate AI model integrity.
- PoW can be used to demonstrate the computational effort put into model training, while PoS can signal the trustworthiness of validators.
Incorporating blockchain into AI workflows can significantly enhance transparency and accountability, addressing bias, data privacy, and algorithmic opacity concerns.
This combination promotes responsible AI development and fosters trust among users, data providers, and regulators. However, it’s essential to consider the scalability, energy efficiency, and regulatory aspects when implementing blockchain in AI systems.
Blockchain’s Role in Enhancing AI Model Trust
Blockchain enhances trust in AI models by providing a secure and transparent framework for AI development and deployment. Here’s how blockchain contributes to improving confidence in AI models:
- Data Provenance and Integrity
- Immutable Audit Trail
- Consensus Mechanisms
- Decentralization
- Smart Contracts for Model Agreements
Data Provenance and Integrity
- Blockchain records the origin and history of data used to train AI models. This provenance ensures that data is genuine, unaltered, and from trustworthy sources.
- Users can verify the authenticity and integrity of the training data, which is essential for building trust in AI systems.
Immutable Audit Trail
- Every transaction on a blockchain is recorded in an immutable ledger. This audit trail includes information about model updates, parameter changes, and data modifications.
- This transparency allows users to track changes to the AI model over time, ensuring accountability and preventing unauthorized tampering.
Consensus Mechanisms
- Blockchain’s consensus mechanisms, such as Proof of Work (PoW) and Proof of Stake (PoS), ensure that the network participants agree upon model updates and changes.
- Consensus adds a layer of trust by confirming that updates to AI models are legitimate and follow predefined rules.
Decentralization
- By operating on a decentralized network of nodes, blockchain reduces the risk of a single point of failure or manipulation. This decentralization fosters trust in the reliability of AI systems.
- Users are less reliant on a central authority, which can be susceptible to biases or misuse of power.
Smart Contracts for Model Agreements
- Smart contracts on blockchain platforms like Ethereum enable automated and trustless execution of agreements between parties.
- AI model developers and users can create smart contracts that define AI models’ terms, conditions, and performance metrics. This ensures transparency and accountability in model usage.
Incorporating blockchain technology into AI systems addresses concerns about transparency, accountability, and data integrity, ultimately enhancing trust in AI models. By providing a tamper-proof and auditable environment, blockchain reinforces AI’s ethical and responsible use across various domains.
Challenges and Considerations
Several challenges and considerations must be addressed when integrating blockchain technology into AI to enhance transparency and trust. These challenges span technical, ethical, and regulatory domains. Here are some key challenges and considerations:
- Scalability
- Privacy and Data Protection
- Integration Challenges
- Regulatory and Legal Implications
- Energy Consumption
- Interoperability
- Costs
Scalability
- Blockchain networks, especially those using Proof of Work (PoW) consensus, can face scalability issues when handling the computational demands of AI models, which can be resource-intensive.
- Solutions like sharding and layer 2 scaling are being developed to mitigate scalability concerns.
Privacy and Data Protection
- Balancing the transparency of blockchain with the need for data privacy can be challenging. Storing sensitive data on a public blockchain may not be appropriate due to privacy regulations.
- Privacy-preserving techniques like zero-knowledge proofs or off-chain data storage must be employed to protect user data while maintaining transparency.
Integration Challenges
- Integrating blockchain and AI technologies requires expertise in both domains. Organizations may face challenges in finding or training personnel with the necessary skills.
- Legacy systems and existing data structures may not easily integrate with blockchain, necessitating substantial infrastructure changes.
Regulatory and Legal Implications
- Blockchain and AI are subject to evolving regulatory landscapes. Compliance with data protection laws like GDPR and adherence to financial regulations can be complex.
- Regulatory clarity and frameworks specific to blockchain-AI applications are still emerging and may vary by jurisdiction.
Energy Consumption
- Blockchain networks, particularly those based on PoW, consume significant amounts of energy. This environmental impact is a concern that needs to be addressed.
- Transitioning to energy-efficient consensus mechanisms like Proof of Stake (PoS) can mitigate energy consumption issues.
Interoperability
- Different blockchain platforms may have their own standards, protocols, and smart contract languages, making it challenging to ensure interoperability and compatibility with AI systems.
- Efforts to create standardized interfaces and protocols are ongoing to facilitate interoperability.
Costs
- Implementing and maintaining blockchain technology can be costly. Organizations must consider the expenses associated with network participation, smart contract development, and ongoing maintenance.
- Cost-benefit analyses should be conducted to determine the feasibility of blockchain integration.
Addressing these challenges and considerations is essential for the successful integration of blockchain technology to enhance transparency and trust in AI models. Collaboration among technologists, regulators, and stakeholders is crucial to navigate these complexities and promote responsible and trustworthy blockchain-AI applications.
Case Studies
Examining real-world case studies provides valuable insights into how blockchain technology can enhance transparency and trust in AI models. Here are a few notable examples:
- Ocean Protocol – Data Marketplace for AI
- SingularityNET – Decentralized AI Marketplace
- IBM Food Trust – Supply Chain Transparency
- Aergo – Decentralized AI Model Deployment
Ocean Protocol – Data Marketplace for AI
-
- Ocean Protocol is a decentralized data marketplace that leverages blockchain to facilitate data sharing while maintaining data privacy and security.
- Data providers can list their data sets on the marketplace, and AI developers can access and purchase them using Ocean tokens.
- Blockchain ensures data provenance, allowing AI developers to trace the source and history of the data, enhancing trust in the quality and authenticity of the training data.
- Smart contracts govern data access and usage agreements, providing transparency and trust for all parties involved.
SingularityNET – Decentralized AI Marketplace
-
- SingularityNET is a decentralized platform that connects AI developers and users, allowing them to buy and sell AI services and models.
- The platform uses blockchain to create a transparent and decentralized AI marketplace where AI agents can interact and collaborate autonomously.
- Smart contracts enable automatic payments and agreements between AI service providers and users, ensuring transaction trust and accountability.
- The transparency of blockchain enhances trust in the AI services and models available on the platform.
IBM Food Trust – Supply Chain Transparency
- IBM Food Trust employs blockchain technology to enhance transparency and traceability in the food supply chain.
- AI algorithms are used to analyze data from sensors, IoT devices, and other sources to track the journey of food products from farm to table.
- Blockchain records each supply chain step, including temperature, location, and handling conditions, ensuring data integrity and transparency.
- Consumers can scan QR codes on food products to access detailed information about their origin, enhancing trust in the safety and authenticity of the food they consume.
Aergo – Decentralized AI Model Deployment
- Aergo is a blockchain platform that enhances transparency and security in AI model deployment.
- Smart contracts on Aergo enable the transparent deployment of AI models, ensuring that models’ behavior adheres to predefined rules and guidelines.
- Users can verify the authenticity and integrity of AI models’ code and data, building trust in the models’ functionality.
- Aergo’s decentralized approach reduces the risk of centralized control and bias in AI deployment.
These case studies illustrate how blockchain technology is applied to diverse domains to enhance transparency, traceability, and trust in AI models and data. They showcase blockchain’s potential to revolutionize how AI is developed, deployed, and trusted in various industries.
Future Trends and Possibilities
The intersection of blockchain and AI holds tremendous promise for the future, with several emerging trends and possibilities that can reshape the way we build, trust, and use AI models. Here are some key future trends and possibilities:
- Enhanced AI Model Explainability
- Privacy-Preserving AI
- Interoperable Blockchains
- Decentralized AI Governance
- Tokenization of AI Models
- AI for Smart Contract Verification
Enhanced AI Model Explainability
Blockchain can enable more granular transparency by recording detailed information about AI model decisions. This can enhance AI model explainability, making understanding and interpreting how AI arrives at its conclusions easier.
Privacy-Preserving AI
Privacy concerns are growing, and combining blockchain with advanced cryptographic techniques will enable AI to process sensitive data without compromising privacy. This is crucial for healthcare, finance, and other sectors.
Interoperable Blockchains
The future may see the development of standards and protocols that allow different blockchains to interoperate seamlessly. This could facilitate the sharing of AI models, data, and services across multiple blockchain networks.
Decentralized AI Governance
Blockchain can support decentralized AI governance mechanisms, where a distributed network makes decisions about AI model behavior and updates of stakeholders. This can enhance fairness and reduce centralized control.
Tokenization of AI Models
AI models could be tokenized on blockchain platforms, allowing users to own, trade, and invest in AI models. This tokenization could democratize AI access and incentivize model development.
AI for Smart Contract Verification
AI can be used to verify the correctness and security of smart contracts on blockchain networks. This can reduce vulnerabilities and enhance trust in blockchain applications.
The future of blockchain and AI holds immense potential for innovation and transformation across industries. As these technologies evolve and mature, they will likely become increasingly intertwined, offering new solutions to complex problems while addressing concerns about transparency, trust, and security in AI systems.
Conclusion
The convergence of blockchain technology and artificial intelligence presents a compelling synergy that addresses critical challenges related to transparency and trust in AI models. This union is poised to shape the future of AI development and deployment profoundly.
As these technologies continue to evolve and mature, they have the potential to revolutionize AI adoption and trustworthiness.
Blockchain’s role in enhancing transparency and trust in AI models will lead to more reliable and accountable AI systems and pave the way for innovative solutions in various domains, ultimately benefiting society.
The future holds great promise as we navigate the exciting intersection of blockchain and artificial intelligence.