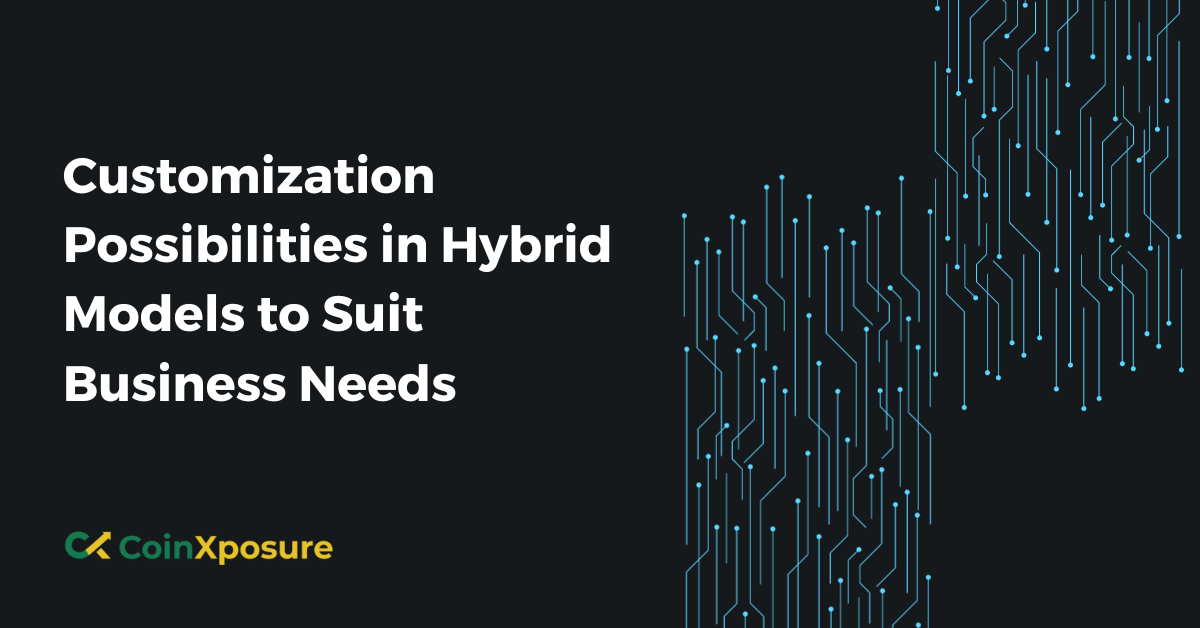
Customization Possibilities in Hybrid Models to Suit Business Needs
In the dynamic landscape of artificial intelligence, the intersection of flexibility and performance is crucial for addressing diverse business challenges.
This article delves into the realm of customization possibilities within hybrid models, exploring how the fusion of different machine learning paradigms offers a tailored approach to meet specific business needs.
As businesses increasingly rely on sophisticated models, understanding the avenues for customization becomes pivotal in optimizing outcomes and ensuring alignment with unique industry requirements.
Types of Hybrid Models
Hybrid models seamlessly blend diverse machine learning techniques to harness the strengths of each component. Three prominent types of hybrid models include:
- Combination of Machine Learning and Rules-based Systems
- Ensemble Models with Various Algorithms
- Integration of Supervised and Unsupervised Learning
Combination of Machine Learning and Rules-based Systems
- Integrates machine learning algorithms with predefined business rules.
- Leverages the interpretability of rules alongside the adaptability of machine learning.
Ensemble Models with Various Algorithms
- Harnesses the power of multiple algorithms to enhance predictive accuracy.
- Examples include Random Forests, Gradient Boosting, and stacking models.
Integration of Supervised and Unsupervised Learning
- Merges labeled and unlabeled data to uncover hidden patterns.
- Balances the strengths of supervised learning for labeled data and the exploratory nature of unsupervised learning.
Customization Dimensions
Customization in hybrid models spans various dimensions, allowing tailored adaptation to specific business contexts:
- Data Input and Preprocessing
- Model Architecture
- Hyperparameter Tuning
- Feature Engineering
Data Input and Preprocessing
- Tailoring data sources to align with business requirements.
- Fine-tuning preprocessing steps for optimal model input.
Model Architecture
- Adapting neural network layers and structures to suit business needs.
- Incorporating domain-specific rules within the model design.
Hyperparameter Tuning
- Optimizing parameters like learning rates and batch sizes.
- Making business-driven adjustments for improved model performance.
Feature Engineering
- Creating domain-specific features for enhanced model understanding.
- Selecting and prioritizing features relevant to the business context.
These dimensions collectively offer a comprehensive framework for customizing hybrid models and tailoring them to the intricacies of specific industries or organizational requirements.
Business-Driven Customization
Business-driven customization in hybrid models involves aligning model design and functionality with the specific needs and goals of a particular industry or organization. Key aspects include:
- Industry-specific Considerations
- Decision-making Criteria
Industry-specific Considerations
- Adapting models to the nuances of sectors such as healthcare, finance, or retail.
- Tailoring algorithms to address unique challenges within a specific industry.
Decision-making Criteria
- Ensuring model outputs align with broader business objectives.
- Incorporating interpretability features to facilitate understanding and trust among stakeholders.
By prioritizing these aspects, business-driven customization ensures that hybrid models not only meet technical benchmarks but also serve as effective tools for solving real-world problems within a given business context.
Challenges in Customization
Customizing hybrid models, while advantageous, poses several challenges:
- Data Quality and Availability
- Computational Resources
- Model Complexity and Interpretability
Data Quality and Availability
- Inconsistent or poor-quality data may hinder effective customization.
- Limited availability of relevant data for specific business use cases can impede model tailoring.
Computational Resources
- Customization often demands increased computational power.
- Resource constraints may limit the exploration of diverse model architectures and hyperparameters.
Model Complexity and Interpretability
- Balancing model complexity for improved performance with the need for interpretability.
- Highly customized models might become challenging to interpret and explain to non-technical stakeholders.
Navigating these challenges requires a thoughtful approach, emphasizing the importance of data governance, resource planning, and maintaining a transparent dialogue between data scientists and business stakeholders.
Future Trends
Future trends in customization of hybrid models are anticipated to revolve around:
- Advancements in Hybrid Model Architectures
- Integration of Explainable AI in Customization
Advancements in Hybrid Model Architectures
- Evolution of more sophisticated fusion techniques for combining diverse learning paradigms.
- Continued research into architectures that seamlessly integrate rule-based systems with advanced machine learning models.
Integration of Explainable AI in Customization
- Growing emphasis on developing hybrid models that not only perform well but also provide clear explanations for their decisions.
- Increased adoption of explainable AI techniques to enhance transparency and build trust in customized models.
As technology progresses, these trends are poised to shape the landscape of hybrid models, making them more adaptable, interpretable, and aligned with the evolving needs of businesses across various industries.
Conclusion
Tthe customization possibilities within hybrid models offer a dynamic and tailored approach to address the intricate challenges of diverse business landscapes.
The fusion of different machine learning paradigms allows for a nuanced adaptation, optimizing models to meet specific industry needs. Navigating dimensions such as data preprocessing, model architecture, hyperparameter tuning, and feature engineering provides a comprehensive framework for customization.
Business-driven customization, anchored in industry-specific considerations and decision-making criteria, ensures that hybrid models not only perform effectively but also align with overarching business objectives.
Despite the promises, challenges such as data quality, computational resources, and maintaining model interpretability underscore the need for careful navigation.
Looking ahead, future trends point towards the continuous evolution of hybrid model architectures and a heightened focus on explainable AI, promising more sophisticated, adaptable, and transparent models.
As organizations strive to leverage the power of AI, a judicious balance between customization and practical constraints will be key to unlocking the full potential of hybrid models in addressing the unique demands of businesses.